

The discovery of more photosynthesis-related genes could help broaden our knowledge of photosynthesis and further help to improve photosynthetic efficiency in plants, especially in crops. Photosynthesis plays a vital role in living organisms on our planet, powering our ecosystem by providing carbohydrates and oxygen. Therefore, we believe it is worth testing the performance of a supervised machine learning approach in predicting the putative biological functions of these “not assigned” genes. This approach was recently successfully tested in maize to predict the functional annotations of non-homology-based genes. Supervised machine learning approaches have recently been rapidly developed in the field of biological applications e.g., AlphaFold, a novel machine learning approach, can predict protein structures with high accuracy. In maize, approximately 9520 genes were sorted to the category of “not assigned” in the last version of MapMan Mercator 4, and we were curious whether there are other ways to help predict the potential functions of these genes. However, these functional categories are based on BLAST sequence similarity and protein domains from InterPro and the Conserved Domain Database (CDD), which assign genes that do not show high sequence similarity to Arabidopsis or contain no typical protein domains to the category of “not assigned”.
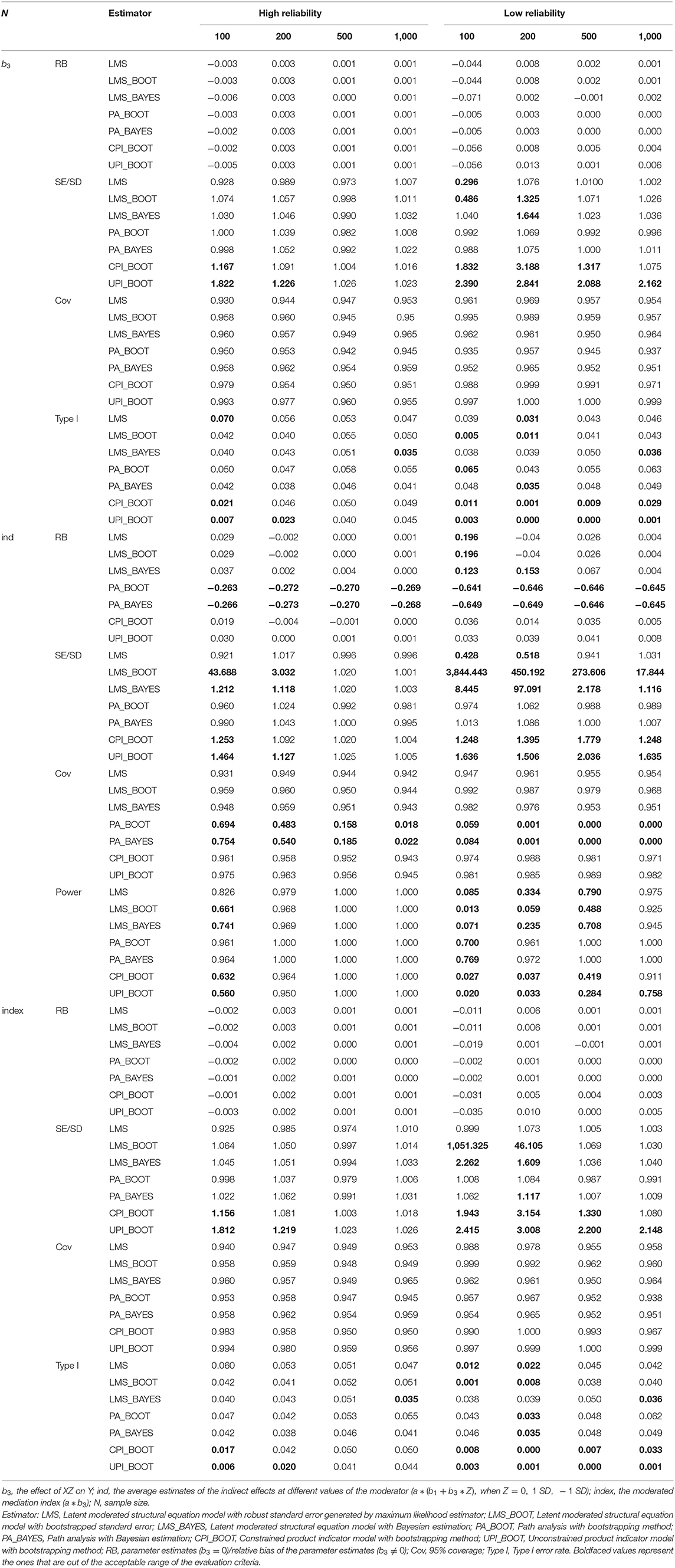
This study reveals a new approach for mining novel genes related to a specific functional category and provides candidate genes for researchers to experimentally define their biological functions.Īnnotations of MapMan functional categories play a pivotal role in helping researchers identify candidate genes. And we put this approach online base on google colab. The protein localization prediction (TargetP) and expression trends of these genes from maize leaf sections indicated that the prediction was reliable and robust. Finally, we predicted 716 photosynthesis-related genes from the “not assigned” category of maize MapMan annotation. And we call this approach “A Machine Learning-based Photosynthetic-related Gene Detection approach (PGD)”. Based on this evaluation, we implemented an ensemble based ML(Machine Learning) methods using a majority voting scheme and observed that including RNA-seq data from multiple photosynthetic mutants rather than only a single mutant could increase prediction accuracy. In this study, we proved the ensemble learning model using a voting eliminates the preferences of single machine learning models. The fast-increasing usage of the machine learning approach in solving biological problems provides us with a new chance to identify novel photosynthetic genes from functional “not assigned” genes in maize.
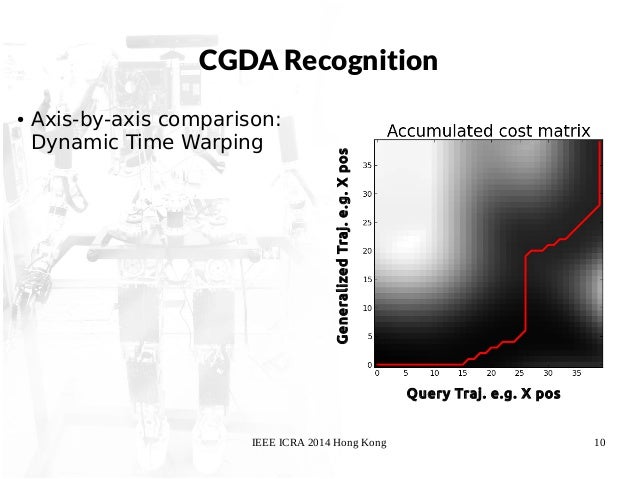
MapMan Mercator 4 is a powerful annotation tool for assigning genes into proper functional categories however, in maize, the functions of approximately 22.15% (9520) of genes remain unclear and are labeled “not assigned”, which may include photosynthesis-related genes that have not yet been identified. Therefore, the mining of genes involved in photosynthesis is important for the study of photosynthesis. The primary determinant of crop yield is photosynthetic capacity, which is under the control of photosynthesis-related genes.
